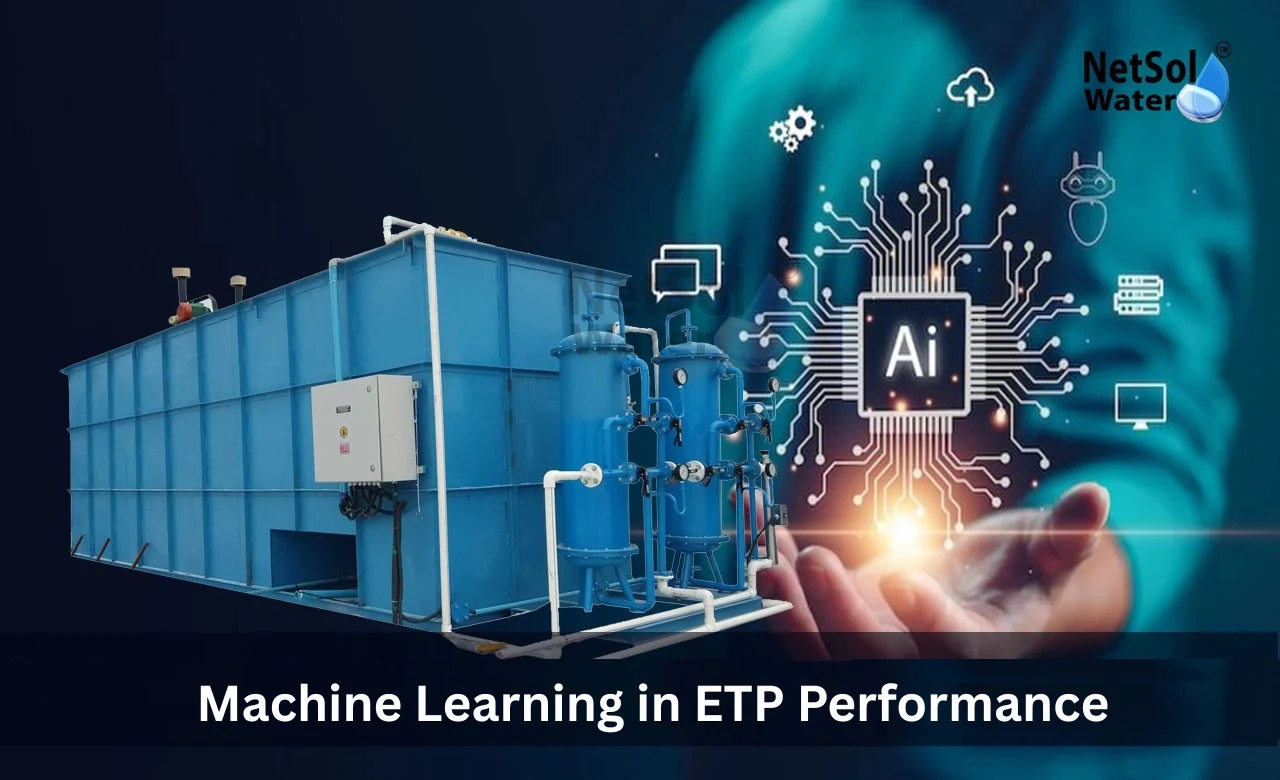
Machine Learning in ETP Performance
Machine Learning brings revolutionary changes to Enhanced Treatment Plant (ETP) operations. This technology enables treatment plants to process waste more effectively through automated monitoring and real-time adjustments. ETPs face growing pressure to meet strict environmental standards while managing increasing waste volumes. Machine learning addresses these challenges by analyzing operational data to optimize treatment processes. The technology learns from historical performance patterns to predict issues before they occur. This predictive capability helps plant operators take proactive steps to maintain optimal performance. Machine learning also adapts treatment parameters based on incoming waste characteristics. This smart approach leads to consistent treatment quality and reduced operational costs. The integration of machine learning in ETPs represents a significant step toward sustainable industrial waste management.
We will explore how machine learning enhances ETP operations through improved monitoring, automated control and predictive maintenance.
Machine Learning Integration in ETPs
The implementation of machine learning in ETPs marks a shift from traditional control systems to intelligent operations. Machine learning algorithms process data from multiple sensors throughout the treatment system. These sensors track parameters such as pH levels dissolved oxygen content and chemical concentrations. The collected data trains the machine learning models to understand normal operating conditions. This understanding enables the system to detect deviations that might affect treatment efficiency. The technology also considers external factors like temperature and flow variations that impact treatment processes. Machine learning systems analyze these complex interactions to maintain optimal treatment conditions. This analytical capability extends beyond basic monitoring to provide actionable insights for plant operators. The integration process involves setting up robust data collection systems and implementing appropriate machine learning models. These models continue to learn and improve their predictions as they process more operational data. This ongoing learning ensures the system becomes more effective at managing ETP operations over time.
Data Collection and Processing
Machine learning systems in ETPs start with comprehensive data collection across treatment stages. Sensors placed at strategic points measure water quality parameters flow rates and equipment performance metrics. The system processes this raw data to identify patterns and relationships between different parameters. Advanced algorithms clean and organize the data to ensure accurate analysis. This processed data forms the foundation for developing predictive models and control strategies.
Model Development and Training
The development of machine learning models involves selecting appropriate algorithms for specific ETP applications. These models undergo training using historical operational data to recognize patterns and make predictions. The training process includes validation steps to ensure model accuracy and reliability. Regular model updates incorporate new data to improve prediction accuracy and adapt to changing conditions.
Optimizing Treatment Processes
Machine learning transforms how ETPs handle treatment processes through intelligent control systems. The technology enables dynamic adjustments to treatment parameters based on real-time conditions. This adaptive approach ensures consistent treatment quality despite variations in influent characteristics. Machine learning algorithms analyze treatment effectiveness and suggest modifications to improve performance. The system learns from successful treatment outcomes to refine its control strategies. This optimization leads to improved treatment efficiency and reduced resource consumption.
Chemical Dosing Control
Machine learning systems manage chemical dosing by analyzing influent characteristics and treatment requirements. The technology predicts optimal chemical doses based on incoming waste properties and desired treatment outcomes. This precise control prevents chemical overdosing and ensures effective treatment. The system adjusts dosing rates in response to changing waste characteristics and treatment conditions.
Energy Management
The application of machine learning in energy management focuses on optimizing power consumption across ETP operations. The system identifies opportunities for energy savings by analyzing equipment performance patterns. Machine learning algorithms schedule equipment operation to minimize energy use during peak demand periods. This smart energy management reduces operational costs while maintaining treatment effectiveness.
Predictive Maintenance and Asset Management
Machine learning enhances ETP maintenance through predictive analytics and asset monitoring. The technology tracks equipment performance metrics to identify potential issues before failures occur. This predictive approach enables planned maintenance activities that minimize disruptions to treatment operations. Machine learning systems analyze equipment vibration patterns temperature changes and performance data to assess asset health. The technology helps maintenance teams prioritize repairs and replacements based on actual equipment conditions. This data-driven maintenance strategy extends equipment life and reduces unexpected breakdowns.
Equipment Health Monitoring
The system continuously monitors critical equipment parameters to assess operational health. Machine learning algorithms analyze these parameters to detect subtle changes that might indicate developing problems. This early detection capability enables maintenance teams to address issues before they affect treatment operations. The technology also tracks maintenance history to improve future maintenance planning and execution.
Performance Prediction
Machine learning models predict future equipment performance based on current operating conditions and historical data. These predictions help operators plan maintenance activities and resource allocation. The system identifies trends that might lead to performance degradation or equipment failure. This predictive capability enables proactive maintenance planning and optimal asset management.
Transform your ETP performance with machine learning solutions
Our team offers expertise in implementing machine learning systems tailored to your treatment plant requirements. Contact us to discover how machine learning can optimize your ETP operations enhance treatment efficiency and reduce operational costs. Schedule a consultation today to start your journey toward smarter ETP management.
To explore customised commercial RO plants, Industrial RO plant, ETP or STP solutions for your needs in your areas and nearby regions, Contact Netsol Water at:
Phone: +91-965-060-8473
Email: enquiry@netsolwater.com